27.2.-3.3.2023 Helsinki/Espoo, Finland
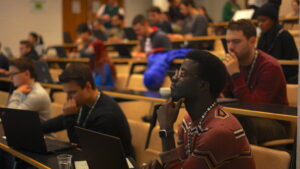
From 27th to 3rd March 2023 the Actively Learning Materials Science workshop was held at Aalto University in Helsinki/Espoo, Finland. This workshop welcomed 81 in-person participants from 10 countries (and many more among the 50+ online participants), also comprising 12 invited members among lecturers, teaching assistants, organizers and technical helpers. The event was sponsored jointly by CECAM, the Psi-k organization, Aalto University, and the Finnish Center for Artificial Intelligence, with talk and poster prizes sponsored by Wiley.
The workshop was dedicated to active learning (AL) algorithms, i.e. algorithms where machine learning datasets are collected on-the-fly in the search for optimal solutions. Paradigmatic examples in this area include (but are not limited to) Active Learning methods, Reinforcement Learning protocols, and Bayesian Optimization approaches. In the tutorials, talks and poster presentations, the participants showcased how AL enables to tackle outstanding problems in the optimal design of experiments, efficient traversal of complicated search spaces for electronic structure simulations and high throughput screening.
A key strength of AL techniques lies in the automated manner in which the machine learning model selects the data to include into the dataset via acquisition strategies. The requested data points can then be evaluated via computation or experiment and included into the model iteratively, until the optimal solution converges. The resulting compact, maximally informative datasets make AL particularly suitable for applications where data is scarce or data acquisition expensive. In this way, AL has helped accelerate materials discovery away from big-data and free of human bias. Despite recent successes, future applications of AL on experimental data are slow, given that key data infrastructure is still lacking. Working with multiple objectives, or multidimensional data remains challenging. Novel method development across the research field is needed to advance AL techniques and associated frameworks in materials research.
Actively Learning Materials Science (AL4MS) focussed on two key objectives, both from a pedagogical (first part of the event) as well as from an advanced perspective (second part of the event): 1) How could data infrastructures and AL algorithm development advance experimental materials discovery? 2) How could we combine multiple channels of information in the same AL model? Continue reading Actively Learning Materials Science Workshop 2023