27.2.-3.3.2023 Helsinki/Espoo, Finland
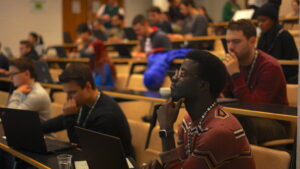
From 27th to 3rd March 2023 the Actively Learning Materials Science workshop was held at Aalto University in Helsinki/Espoo, Finland. This workshop welcomed 81 in-person participants from 10 countries (and many more among the 50+ online participants), also comprising 12 invited members among lecturers, teaching assistants, organizers and technical helpers. The event was sponsored jointly by CECAM, the Psi-k organization, Aalto University, and the Finnish Center for Artificial Intelligence, with talk and poster prizes sponsored by Wiley.
The workshop was dedicated to active learning (AL) algorithms, i.e. algorithms where machine learning datasets are collected on-the-fly in the search for optimal solutions. Paradigmatic examples in this area include (but are not limited to) Active Learning methods, Reinforcement Learning protocols, and Bayesian Optimization approaches. In the tutorials, talks and poster presentations, the participants showcased how AL enables to tackle outstanding problems in the optimal design of experiments, efficient traversal of complicated search spaces for electronic structure simulations and high throughput screening.
A key strength of AL techniques lies in the automated manner in which the machine learning model selects the data to include into the dataset via acquisition strategies. The requested data points can then be evaluated via computation or experiment and included into the model iteratively, until the optimal solution converges. The resulting compact, maximally informative datasets make AL particularly suitable for applications where data is scarce or data acquisition expensive. In this way, AL has helped accelerate materials discovery away from big-data and free of human bias. Despite recent successes, future applications of AL on experimental data are slow, given that key data infrastructure is still lacking. Working with multiple objectives, or multidimensional data remains challenging. Novel method development across the research field is needed to advance AL techniques and associated frameworks in materials research.
Actively Learning Materials Science (AL4MS) focussed on two key objectives, both from a pedagogical (first part of the event) as well as from an advanced perspective (second part of the event): 1) How could data infrastructures and AL algorithm development advance experimental materials discovery? 2) How could we combine multiple channels of information in the same AL model?
Outcomes
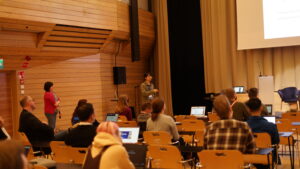
The introductory part of the workshop paved the way to an overview of active learning methods, e.g. Bayesian optimization and reinforcement learning, in the context of atomistic modelling, experiments, and machine learning potential generation. The relevance of FAIR protocols and automated workflows was also highlighted through a dedicated lecture + hands-on session. Tutorials used publicly available open source and documented codes.
The workshop programme focused on active learning approaches for optimising model prediction or target materials properties. Several contributions presented active learning methods with Bayesian optimization or reinforcement learning, but also with decision tree models. Technical discussions on state-of-the-art algorithms featured tailored acquisition functions for Bayesian approaches, and semi-supervised methods to circumvent missing data.
As a natural byproduct of the interest in Bayesian optimization algorithms, the workshop participants explored in rich detail the methods to predict model uncertainty and dataset curation. There was discussion about ensemble models, Bayesian approaches, or approaches based on the statistical/geometrical properties of the training set and the representation used to encode the information in the latter.
In relation to materials chemistry, AL4MS featured diverse applications ranging from energy materials (perovskites and batteries), to metallurgy and chemical reactions. The exploitation of information between experiments and simulations has been considered. There were discussions on how to leverage experimental data to produce better theoretical models, and how to exploit descriptors from simulations to better predict experimental outcomes.
The workshop also highlighted possible emerging research trends: in particular, multi-modality models, which use information from various sources such as text, images, and spectra. We also heard about multi-fidelity models, which harness data from sources with different fidelity levels, such as well-controlled experiments versus less controlled sources like literature. While it is crucial to use reliable and trustworthy data sources for machine learning, the information therein can be (too) little, such as it might also be beneficial to leverage information from multiple sources, so as to train a more informed model.
Throughout the conference, the importance of cross-contamination of expertise was a prominent and recurring theme. Participants voiced the need to foster interactions between communities, by identifying pressing and realistic problems, during panel discussions and presentations. The presence of leaders in atomistic modelling, computer science, machine learning, experimentalists, and industry representatives enabled an interdisciplinary exchange of perspectives and experiences. Our workshop was indeed specifically designed to identify the needs of multi- and interdisciplinary cross-contamination.
All attendees (to our knowledge), including invited speakers, online participants, poster presenters, and young researchers attending their first conference on the topic, have expressed satisfaction with the structure, topics, and organisation of the event. Our feedback collection analysis demonstrated a very high satisfaction overall (4.3/5), with the workshop organisation (4.6/5) and facilities (4.5/5). The workshop was rated as a highly enjoyable experience (4.5/5). We received some comments with suggestions for topics to explore in a future workshop, e.g. “Federated learning, ML for other types of materials data e.g. images, graphs, time series”.
Event Website: https://sites.utu.fi/al4ms2023/
Full program: https://sites.utu.fi/al4ms2023/programme/
Book of Abstracts:
https://sites.utu.fi/al4ms2023/wp-content/uploads/sites/1231/2023/03/AL4MS_2023_AbstractBook.pdf
Full list of participants:
https://sites.utu.fi/al4ms2023/list-of-participants/
Workshop media and session recordings:
https://sites.utu.fi/al4ms2023/media-and-tutorials/
Community needs
The computational needs in machine-learning for materials science are twofold: 1) data generation and 2) machine-learning model training and evaluation. For 1), data is typically generated with electronic structure theory methods (e.g. density-functional theory). The electronic structure theory community is well established with mature methods and codes. Electronic structure theory is resource intensive, however, and requires access to large HPC resources, in particular, because machine-learning datasets are large. For 2), machine learning codes are typically not as complex as electronic structure theory codes. They also frequently utilize established machine-learning libraries like scikit learn, pytorch or tensorflow. Machine-learning training can be costly (e.g. large matrix inversion in kernel methods or time intensive neural network training) and is frequently carried out on GPUs. Easy access to GPUs would facilitate model training.
The AL4MS purposefully widened the scope and brought together a diverse set of speakers from machine-learning method development, experiment and a variety of application domains. While machine-learning is progressing rapidly in computational materials science, experimental materials science is slower to catch on and data is scarcer and more heterogeneous. AL4MS reached out to experimental work in e.g. biomaterials, photovoltaics and battery materials, but more outreach and networking of this kind is required, and will be pursued, in the future.
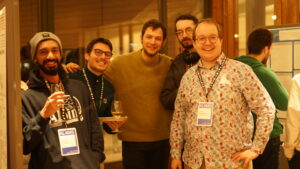
Scientific, Technologic, and Societal Impact
The discovery of new materials for use in catalytic applications, energy storage, diagnostics, and therapeutics is crucial for achieving sustainable and equitable development, as outlined by the UN Sustainable Development Goals (SDGs). All participants agreed on the urgency of the development objectives.
In recent years, there has been a surge in the development and application of machine learning technologies in materials science, yielding promising results across a range of applications. These include the design of sustainable reaction conversion and manufacturing processes, high-performance materials for green energy (such as photovoltaics) and transport (such as lightweight robust metals). While significant strides have been made in algorithm design and theoretical understanding, large-scale real-world applications are only beginning to emerge, leading to the discovery of new stable materials and the design of never-seen-before materials.
AL4MS advanced research in these critical fields by disseminating the latest research advancements to young researchers and fostering collaboration among renowned scientists from diverse fields. By equipping the next generation of scientists with the skills to address complex problems related to high-performance materials design, we aim to further drive progress in these areas.
In the workshop we highlighted the transfer of knowledge from academic research into the industry, promoting technological impact. Industry representatives from Microsoft Research, Toyota Research, and Outokompu provided participants with insights into possible career pathways while offering an overview of the state-of-the-art methods and achievements within industrial R&D. These novel developments are set to boost innovative technologies and create societal impact.