Held at Zuse Institute, Berlin, July 8-12, 2024
On 8-12 of July 2024, we gathered at the CECAM node at the Zuse Institute Berlin to delve into the topic of Machine Learning of First Principles Observables. Seventy-five participants travelled to attend the event in person and nearly one hundred registered to join remotely. The event was jointly sponsored by CECAM, the Psi-k Charity, Deutsche Forschungsgemeinschaft, and the Max-Planck-Gesellschaft.
The workshop addressed the growing need for models, workflows, and databases that go beyond the established methods of producing machine learning (ML) interatomic potentials and serve to predict experimentally observable quantities. During the event, we addressed the topic in eight subject-specific sessions, each consisting of four talks and a panel discussion, which covered topics from “Thermodynamic observables” to “Long-range interactions” and “Spectroscopy” and considered the future advancements of the field. The invited and contributed speakers came from a range of career stages, from both theoretical and experimental backgrounds.
Main takeaways
The eight sessions of the workshop were focusing on the following topics:
– Thermodynamic Observables
– Electronic Structure and Long-Range Interactions (3 sessions)
– Magnetic Observables
– Spectroscopic Observables (2 sessions)
– Databases and Reaction Networks
Overarching all sessions, several topics were identified to be very important in forming this community:
Data Sharing and Management: In almost every panel discussion, the importance of effective data sharing, meta-data utilisation, and the creation and maintenance of curated databases was discussed. It was also emphasised that these databases should also include negative results, which further constrain the ML models and make them more robust. These data are critical for experimentally relevant ML models for the future. The importance of code documentation and reproducibility was also highlighted during the discussions.
Bridging Experiment and Simulation: This workshop served as a springboard for facilitating exchange between theoreticians and experimentalists. By encouraging discussion between both groups, the speakers and participants identified several areas where these two groups could bridge the multi-scaling gap from both ends. This involves theoreticians reconsidering the approximations and simplifications in their models to make them more realistic by incorporating factors such as interfaces and defects. At the same time, experimentalists were encouraged to conduct repeated experiments on less complex model systems whose simulations are more attainable for the current computational approaches. This dual approach aims to bring theory and experiment closer together, bridging the complexity gap from both ends.
Metrics for Evaluating Predicted Data: The final topic that emerged during the workshop was the need for better metrics for evaluating the accuracy of the predicted data beyond simple scalar values. The discussion covered metrics which allow for tolerance in variations in spectra shifts, peak width, and spectral intensities. Additionally, the importance of general and foundational ML models such as MACE-MP-0, ChargNET and AIMNet2 , as well as the need for benchmarking them in more realistic atomistic modelling tasks, was highlighted.
Workshop format
Each of the morning or afternoon sessions consisted of an invited overview talk, three invited or contributed talks, and a panel discussion led by the session chair. While the oral presentations are essential to every workshop, the addition of generous panel discussions allowed for some time to reflect, debate, and elucidate on the session topics in the bigger picture, and also engaged a good number of the audience members. The discussions continued throughout the week during the poster session on Monday, workshop dinner on Wednesday and walking tours on Thursday, allowing for the participants to connect in a less formal setting.
It was important to us to open the workshop up for remote participation, to reach and include those we could not accommodate due to capacity limitations or who could not join onsite. The most significant remote aspect was streaming the talks over Zoom where around 50 participants joined each of the sessions.
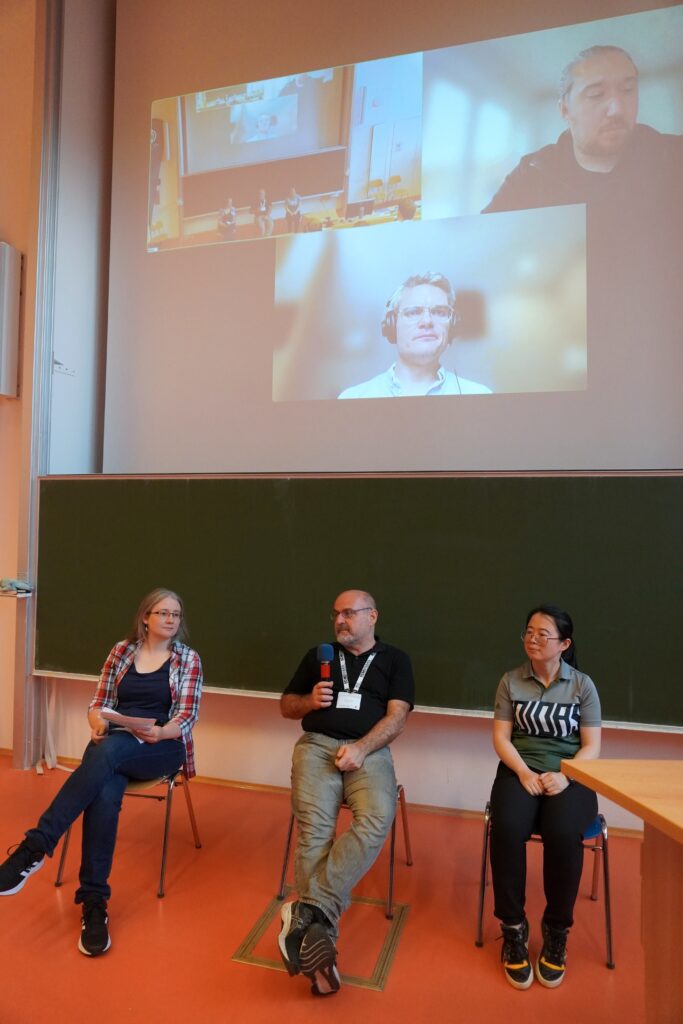
Detailed programme
- Thermodynamic Observables
- Prof. Dr. Karsten Reuter: Out of the Crystalline Comfort Zone: Tackling Working Interfaces with Machine Learning
- Dr. Michele Simoncelli: Machine learning opens a wonderland for looking through glasses
- Dr. Christian Carbogno: Accelerating Transport Coefficient Predictions via Machine Learning
- Prof. Dr. Nong Artrith: Harnessing Machine Learning for Advancing Amorphous Battery Materials
- Electronic Structure & Long-Range Interactions I
- Prof. Dr. Gábor Csányi: A foundational atomistic model for materials
- Prof. Dr. Janine George: High-throughput Approaches for Materials Understanding and Design
- Sergey Pozdnyakov: Challenging the dogma of rotational equivariance in atomistic ML
- Prof. Dr. Kulbir Ghuman: Leveraging Computational Advances to Design and Optimize Energy Materials: From Traditional Methods to Machine Learning
- Electronic Structure & Long Range Interactions II
- Prof. Dr. Michele Ceriotti: Machine-learning for electronic structure
- Alexander Knoll: Advanced Software Frameworks for Describing Local and Non-Local Interactions in High-Dimensional Neural Network Potentials
- Prof. Dr. Reinhard Maurer: Electronic Structure Surrogate Learning for Quantum Dynamics and Inverse Design
- William Baldwin: ML Electrostatics Models in Relevant Test Systems
- Magnetic Observables
- Prof. Dr. Stefano Sanvito: The Jacobi-Legendre framework for materials discovery
- Johannes Wasmer: Prediction of magnetic exchange interaction in doped topological insulators
- Prof. Dr. Alessandro Lunghi: Machine Learning for Molecular Magnetism
- Shuping Guo: Machine learning facilitated by microscopic features for discovery of novel magnetic double perovskites
- Spectroscopic Observables I
- Prof. Dr. Patrick Rinke: Machine Learning for Spectroscopy – Concepts, Successes, and Challenges
- Dr. Tigany Zarrouk: Experiment-driven atomistic materials modeling: Combining XPS and MLPs to infer the structure of a-COx
- Prof. Dr. Rose Cersonsky: Categorizing three-dimensional photonic crystals: open challenges in scale-covariant problems
- Clelia Middleton: p-DOS: a descriptor with electronic wisdom for learning X-Ray spectroscopy
- Spectroscopic Observables II
- Prof. Dr. Rebecca Nicholls: Interpreting core-loss spectroscopy
- Prof. Dr. Josef Granwehr: Predicting electron paramagnetic resonance parameters and their sensitivity to structural configuration
- Prof. Dr. Claudia Draxl: Assessing spectroscopic features: from fingerprinting to predictions
- Prof. Dr. Stefan Sandfeld: Scientific Machine Learning and Explainable AI Approaches for the Physical Sciences
- Electronic Structure & Long-Range Interactions III
- Luca Leoni: Machine learned small polaron dynamics
- Bartosz Brzoza: Applying SE(3)-Equivariant Attentional Graph Neural Networks for the purpose of predicting the electronic structure of molecular hydrogen
- Databases & Reaction Networks
- Prof. Dr. Johannes Margraf: Machine Learning in Chemical Reaction Space
- Dr. Jonathan Schmidt: Alexandria database: All you need is more data in material science?
- Prof. Dr. Olexandr Isayev: Scaling Molecular Modeling to Millions of Reactions with Neural Network Potentials
- Dr. Pierre-Paul De Breuck: Property predictions from limited and multi-fidelity datasets
Organizers
- Simone Köcher (IET-1, Forschungszentrum Jülich GmbH)
- Angela Harper (Fritz-Haber Institut der Max Planck Gesellschaft)
- Hanna Türk (École Polytechnique Fédérale de Lausanne)
- Elena Gelžinytė (Fritz-Haber Institut der Max Planck Gesellschaft)
- Giulia Glorani (Fritz-Haber Institut der Max Planck Gesellschaft)
Further details
- Workshop booklet;
- Workshop website, which includes the abstracts and the full list of participants.